AI Assurance Framework
Your guide to using and operating AI systems.
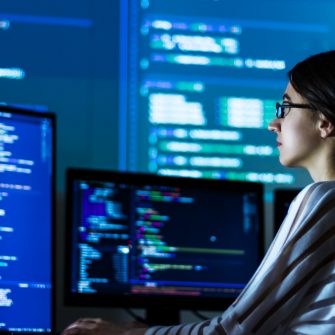
What is the AI Self-Assurance Framework?
The UNSW AI Ethical Assurance Framework aims to establish a centralised self assessment approach AI deployment or development. The framework has been developed for use when considering the use of an AI system. It is intended to provide guidance when designing, building and operating AI systems.
When contemplating the deployment of a particular AI technology, each of the 7 parts of the self-assurance framework should be used to guide decision making. This will support UNSW to innovate using AI systems safely and in accordance with the Ethical and Responsible Use of Artificial Intelligence at UNSW. Each part has an explanatory overview and key questions that should be considered.
The Self-Assurance Framework should be used to consider whether to proceed with the development or deployment of an AI system.
What factors should you consider in the assurance framework?
By using advanced security features, Microsoft Copilot maintains the confidentiality and integrity of sensitive business information. It adheres to compliance standards, safeguards against unauthorised access and implements industry-leading encryption methods to protect your commercial data.
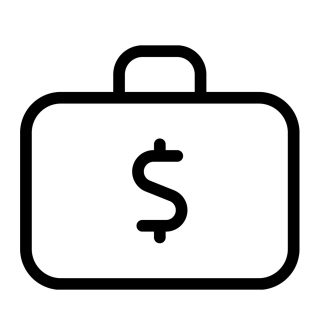
Business value
The proposed solution must align with UNSW’s Core Values and Strategic Plan and improve services or efficiencies. Approval for the project and related expenses, such as software development and maintenance, must be granted by the relevant budget owner or sponsor. Key review points include:
UNSW Vision: To improve lives globally through innovative research, transformative education and commitment to a just society, as outlined in the UNSW 2025 Strategy.
UNSW Values:
Demonstrates Excellence: Delivers high performance and service excellence.
Embraces Diversity: Values individual differences and promotes inclusion.
Drives Innovation: Thinks creatively and embraces change.
Displays Respect: Treats others with dignity and communicates with integrity.
Builds Collaboration: Works effectively within teams and builds relationships with stakeholders.
Improvement in services or efficiencies should be measurable, aligned with organisational objectives (2024 Strategic Priorities) and attributable to the specific project or technology. Initial and ongoing costs need to be budgeted according to the financial modelling template within the business case process.
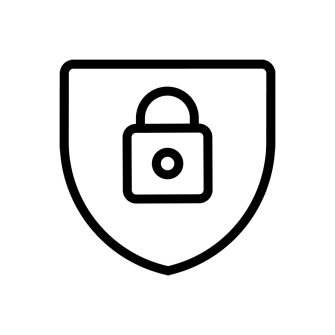
Sensitive data
The key issue to consider is whether the solution involves the use or creation of sensitive, commercial, or personal data.
UNSW is committed to maintaining the security, integrity, and appropriate management of its data. To ensure data is kept secure, it is classified and only used or stored in applications, systems, or platforms with the appropriate level of data protection. This is crucial when engaging with AI systems, as data must be stored in systems that match or exceed the data's classification level.
The UNSW Data Classification Standard defines data as Public, Private, Sensitive, or Highly Sensitive and should be consulted when using AI to analyse or review UNSW data. A comprehensive list of examples is available from Cyber Security.
When sharing UNSW data, even internally, a Data Sharing Agreement (DSA) is required. Sharing Personal Information (classified as Sensitive or Highly Sensitive) always requires a DSA. Additional information is available on the SharePoint page for DSAs.
Additionally, consider the Information Governance policy (still in development) when using UNSW data within AI systems. This will combine current data governance, privacy, recordkeeping, and cybersecurity policies (see Compliance).
Sensitive data considerations may apply when using or creating data about:
- Children
- Religious affiliation
- Race or ethnicity
- Political associations
- Trade union associations
- Gender and/or sexual diversity
- Criminal records
- Health or genetic information
- Other personal data
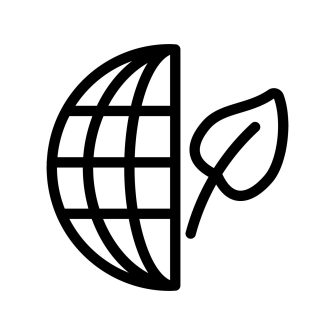
Harm
There is growing public concern about the potential for AI to cause harm. While not all uses of AI impact human rights, AI-informed decision-making can have significant consequences. Key areas of harm to consider include:
- Physical harm
- Psychological harm
- Environmental or community harm
- Unauthorised use of sensitive or personal information
- Unintended identification or misidentification
- Infringement of intellectual property rights
- Financial or commercial impact
- Incorrect advice or guidance
- Inconvenience or delays
AI-informed decision making
The term "AI-informed decision-making" refers to decisions that are significantly assisted by AI technology and have legal or significant effects on individuals. The Australian Human Rights Commission recommends that AI-informed decisions be lawful, transparent, explainable, used responsibly, and subject to human oversight, review, and intervention.
Monitoring and testing
You must closely monitor your AI system for potential harms by assessing outputs and testing results to identify unintended consequences. It is essential to quantify these effects, even during testing and pilot phases, as consequential decisions can cause real harm. Be aware that changes in the AI system's context or usage can lead to unexpected outcomes. Any planned changes should be thoroughly evaluated and monitored.
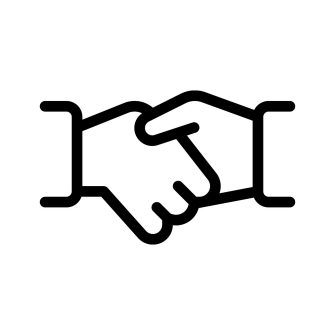
Fairness
Fairness in AI systems requires safeguards to manage data bias and quality risks. For instance, can you explain why you selected specific data for your project over others? Can you justify the data's relevance and permissions? AI systems often draw from multiple datasets to find new patterns and insights, so it's crucial to decide whether you should use the data, especially if historical data was collected for different purposes.
Improving fairness in AI
- Diverse data: Ensure AI systems are trained on diverse and representative data to reduce bias. This helps the system reflect real-world diversity and consider all groups affected by its decisions, including cultural sensitivities and underrepresented populations.
- Bias detection: Use tools and techniques to detect and measure bias in AI systems. Open-source tools like Microsoft’s Fairlearn toolkit offer visualisation dashboards and algorithms to mitigate unfairness.
- Stakeholder engagement: Involve a wide range of stakeholders, especially those affected by the AI system, in the development process to identify potential unfairness and align the system with societal values.
- Ethical principles: Adhere to ethical principles such as fairness, accountability, transparency, and ethics to guide AI development.
- Governance structures: Establish governance structures to oversee AI development and deployment, ensuring fairness is prioritised throughout the system's lifecycle. The World Economic Forum discusses this in more detail.
- Continuous monitoring: Continuously monitor AI systems after deployment using performance measures and fairness targets to identify and correct biases. Determine fairness measures at the scoping stage to prioritise important decisions from the outset.
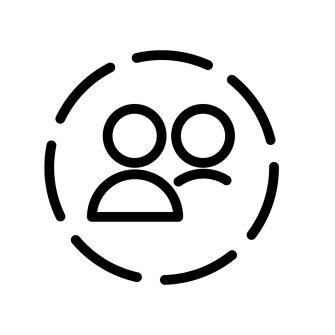
Transparency & accountability
The inner workings of commercial AI systems can be inaccessible and complex, posing risks when relying on unexplainable insights or decisions. To mitigate these risks, consider the role of human judgment in assessing AI outputs.
Assessing AI-generated insights
If you can't explain how an AI system produces its insights, evaluate the potential harms, their likelihood, and the ease of reversing them. Document these considerations, especially if midrange or higher risks are identified.
Consult with the relevant user community when designing AI systems, particularly operational ones. Ensure accountability and transparency, demonstrating positive benefits and outcomes.
Transparency and accountability
Transparency requires that individuals affected by AI decisions understand the basis of these decisions and have the means to challenge them if they are unjust or unlawful. Ensure that no one loses rights, privileges, or entitlements without access to a review process.
You must have a way to explain how AI informs decisions. If the system is a “black box” or too complex to explain, human judgment should be used to intervene before acting on AI-generated insights. Document this oversight process. In low-risk environments, it may be sufficient to have mechanisms to easily reverse actions, like overriding an automated barrier.
Operator training
There is a risk of over-reliance on AI results. Ensure system operators, including those exercising judgment over insights, are trained to critically assess AI-generated outputs and understand system limitations. For operational AI systems, users should be confident they can reverse any harm or that a Responsible Officer can make informed decisions. Non-operational system users must be skilled in interpreting AI insights if they are to be relied upon.
Assigning responsibility
Identify who is responsible for:
- Using AI system insights/decisions
- Project outcomes
- Technical performance of the AI system
- Data governance
These roles should ideally be held by different individuals who are senior, skilled, and qualified.
Human oversight
For operational AI systems, ensure that humans can intervene and are accountable, which may also apply to non-operational systems. This fosters user confidence and control in your AI system.
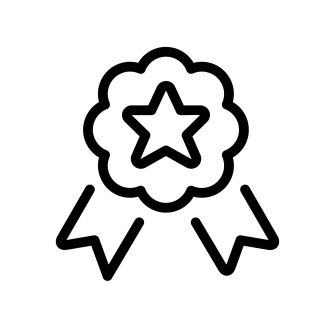
Resilience & safety
Even small AI system projects may have privacy or security vulnerabilities. For example, an analytics project that stores commercially sensitive data in a non-secure environment unbeknown to the user.
As with any emerging technology, AI systems can pose new cyber security risks and so it is important to be vigilant. You must comply with the mandatory requirements as identified in the Cyber Security Policy.
Other critical policies to consider include:
There are standards which provide for better practice in all of our technology developments which remain crucial for AI system developments.
- Cyber Security Standard, Risk Management
- Cyber Security Standard, Data Security
- Data Classification Standard
A critical first step is completing a Vendor Security Risk Assessment to ensure that they meet the minimum criteria necessary to protect UNSW.
For help on assuring your AI system remains cyber compliant please visit MyCyberHub
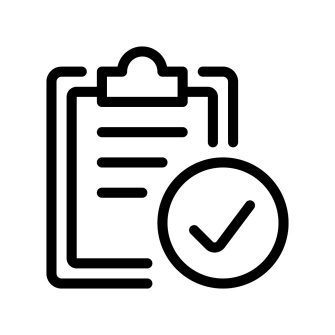
Compliance
Just like everything else that we do, the use of AI must comply with UNSW Policies, the Ethical and Responsible Use of Artificial Intelligence at UNSW, UNSW’s guiding principles on AI, and relevant legislation.
Critical policies to consider include:
- Cyber Security
- Data Breach Policy, Policy & Procedure
- Risk Management Policy
- Data Governance Policy
- Privacy Policy
- IP Policy
This should include relevant standards.
You must also make sure your data use aligns with relevant privacy, discrimination, copyright and government information and records legislation.
In addition, any AI solution proposed to be deployed should align with UNSW Strategic Outcomes as outlined in the Business Value section below. It is also important to consider whether there is an alternative way to deliver the benefit attributed to the solution, without the use of AI.
There are several resources available that can guide our use of AI and help us observe compliance standards. These include Australia’s AI Ethics Principles (Australia’s Artificial Intelligence Ethics Framework).
This is supported by the Group of Eight Principles on the Use of Generative Artificial Intelligence for use by its members within higher education:
Maintaining academic excellence and integrity in teaching, learning, assessment and research will continue to be a priority for Go8 universities as they adapt and lead in the responsible use of generative AI.
Go8 universities will promulgate clear guidelines for the appropriate use of generative AI technologies by their academic staff, researchers and students.
Go8 universities will develop resources to empower students, academic and research staff to engage productively, effectively and ethically with generative AI.
We will work to ensure equal access to generative AI.
Go8 universities will engage in collaborative efforts to exchange and implement best practices as generative AI technology and its role in society continue to advance.
For those participating in research and education in Europe, further resources are available in the EU Briefing on EU Legislation in Progress.
The procurement process may be the best place to ensure the mandatory policy requirements for AI systems are considered early on and measures are taken to mitigate risk in using AI generative tools. Mechanisms for ensuring performance, ongoing monitoring and calibration as well as management of risk may be negotiated and built into contractual agreements with vendors. Please make sure you seek help from procurement experts and appropriate legal support.
AI in context: Real world examples and scenarios
Scenario 1
If an AI system or machine learning makes a quantitative prediction about a student’s likelihood of failing a particular unit based on assessing a range of activities and results. This prediction value creates a new piece of personal information about the student.
In this case, the key elements which need to be considered include:
Scenario 2
When an AI tool makes decisions about a person and action is taken as a result of the decision, and the decision process has no human element to oversee the decision, this would not be considered appropriate. An assessment may be made based on data matching from different sources, and there is a good reason for the discrepancy in data. In this case, the rationale for the discrepancy would need to be reviewed by a human, and an assessment made as to whether the decision made and the corresponding action proposed is valid.
In this case, the key elements which need to be considered include:
Scenario 3
When students are using AI tools to assist (partly or even fully) in completing an assessment task, the onus is placed upon those marking the assessments. Assessors are given a fixed timeframe allocated to review and grade assessment tasks. Assessors can usually ascertain when AI has been used however, the assessor would typically need to pay extremely close attention to identify the indicators that AI was used, perhaps outside of the relevant School’s policies. Even if assessors have been provided with appropriate training to undertake this task, is this a fair requirement to be placed on assessors without review of the current marking constraints? Failure to identify the use of AI means potential (and serious) student conduct events can be missed, which may lead to more instances.
In this case, the key elements which need to be considered include:
Where can I find further information and learning?
-
Responsible AI investments and safeguards for facial recognition | Azure Blog | Microsoft Azure
Microsoft Responsible AI Standard v2 General Requirements
Microsoft-RAI-Impact-Assessment-Template.pdf
GitHub - microsoft/responsible-ai-toolbox: Responsible AI Toolbox is a suite of tools providing model and data exploration and assessment user interfaces and libraries that enable a better understanding of AI systems. These interfaces and libraries empower developers and stakeholders of AI systems to develop and monitor AI more responsibly and take better data-driven actions.
Responsible and trusted AI - Cloud Adoption Framework | Microsoft Learn
Overview of Responsible use of AI - Azure AI services | Microsoft Learn
Responsible Conversational AI Demo - AI Demos (microsoft.com)
-
Digital NSW has some great learning modules:
- A common understanding: simplified AI definitions from leading standards
- Generative AI: basic guidance
- Chatbot prompt essentials
- Cyber Security NSW generative artificial intelligence (AI)
Important: Please ensure you have registered a personal email address or mobile phone number in your myUNSW profile. Otherwise, you will not be able to use the UNSW Identity Manager services and will have to contact the UNSW IT Service Centre for assistance.