P004: Enhancing Enterprise Architecture Modelling
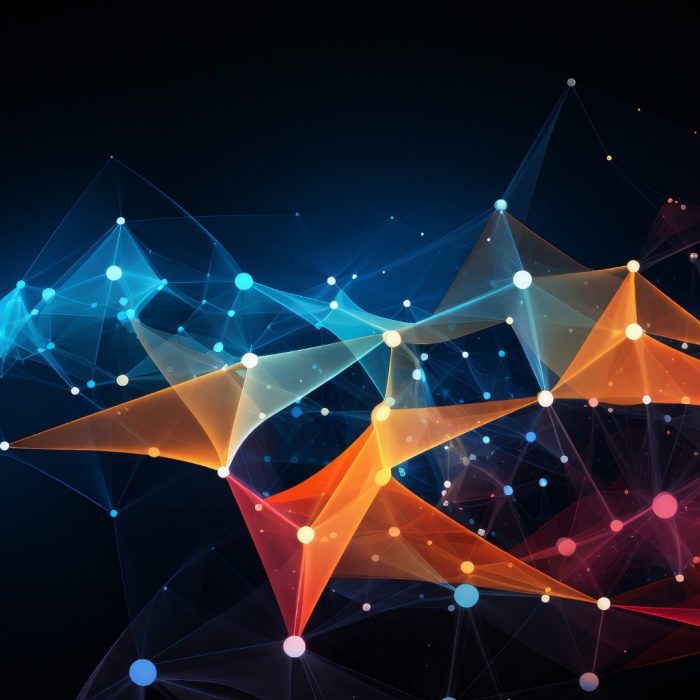
The project aims to explore strategies for improving the quality of answers generated by Large Language Models (LLMs) in response to enterprise-related queries through the use of knowledge graphs to model enterprise and domain-specific ontology.
Organisations often face a range of issues pertaining to the management of enterprise data and information. Thus, critical decisions are made without the right insights out of fragmented knowledge which exist in silos. With the evolution of Generative Artificial intelligence (GenAI), organisationsorganizations are exposed to new opportunities for differentiation and many have been investing on a variety of technologies to enhance the personalisedpersonalized nature of their human-machine touch points while overcoming the knowledge management issues. However, these initiatives seem to lack one of the aspects that is key to enterprise decision making, which is the contextual awareness aligned with the relevant domain ontology.
Enterprise decisions are often backed by contextual awareness and the variability of the connections. In many cases, the relationships among data are more important than the data attributes alone. Conventional (relational) databases capture only the most fundamental relationships among data elements and this amounts to only a fraction of the possible relationships that actually exist. Furthermore, these data models are also embedded with initial assumptions about relationships which are difficult to be revised or assessed.
Graph technologies on the other hand can be initiated with limited and incomplete information less information about data and organisation combined with existing ontologies. Furthermore, the flexibility in modelling makes it possible to accommodate new use cases and data types along the way. Most importantly, their ability to capture inherent relationships among entities facilitates the incorporation of contextual information into business analytics and enterprise architecture solutions. A knowledge graph can be thought of as a machine-readable data structure that represents relationships among heterogeneousheterogenous data sources as nodes and links.
Knowledge graphs help to eliminate several barriers that organisationsorganizations face when it comes to information managementAI adoption such as data complexity, quality and accessibility.
This project will aims to help organisations via the following avenues.
- Exploring how knowledge graphs can leverage the use of unstructured data by linking them with existing using metadata that can be related and managed
- Managing silos of business domain knowledge data where the meaning, usage and consumption patterns of informationdata cannot be precisely defined
- Access to better business insights by identifying bottlenecks in critical business processes and customer segments
Objectives
- Explore novel mechanisms to extract structured content and insights from unstructured business artefacts
- Evaluate the quality of generated content in line with enterprise ontology
- Investigate the possibility of incorporating Conversational Artificial Intelligence to provide guidance for enterprise modelling
-
Fethi Rabhi (Lead - UNSW CSE), Iromie Samarasekara (PhD student), Terry Roach (Founder - Capsifi), Rouzbeh Meymandpour (Mentor - Capsifi), Madhushi Bandara (UTS)
-