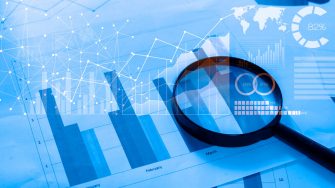
The R package, namely WAvelet System Prediction (WASP), is based on a discrete wavelet transform (DWT)-based variance transformation method (Jiang et al., 2020). This method refines predictor spectral representation using wavelet theory and improves the prediction accuracy of the associated response. We further introduce the maximal overlap DWT (MODWT)-based variance transformation which allows the method to be used in forecasting applications. We also develop the method to include an unbiased estimator which mitigates the well-known issue of edge effects in wavelet transforms. The predictive model in the framework is a k-nearest neighbor (knn) approach. The main functionalities of the software include: (1) transforming the system predictors, (2) identifying significant predictors corresponding to the response, (3) predicting target response using the knn. An application to predicting sustained drought anomalies across Australia in the EMS paper shows clear improvements in predictive skill compared to the use of untransformed predictors.
Refrences
- Jiang, Z., Rashid, M. M., Johnson, F., & Sharma, A. (2020). A wavelet-based tool to modulate variance in predictors: An application to predicting drought anomalies. Environmental Modelling & Software, 104907. doi:https://doi.org/10.1016/j.envsoft.2020.104907
- Jiang, Z., Sharma, A., & Johnson, F. (2020). Refining Predictor Spectral Representation Using Wavelet Theory for Improved Natural System Modeling. Water Resources Research, 56(3), e2019WR026962. doi:https://doi.org/10.1029/2019WR026962