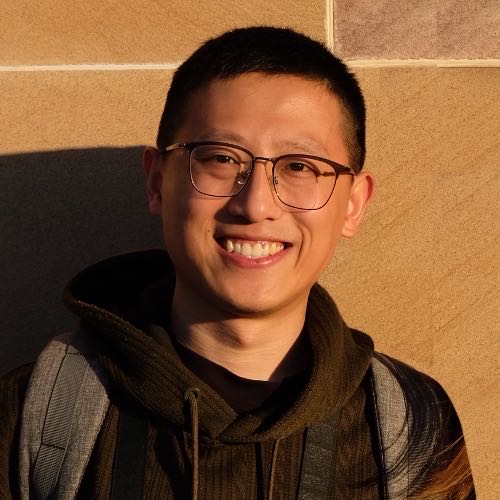
Dr Yongjing Mao
PhD | 2022 |
Remote Sensing and Coastal Geomorphology, The University of Queensland, Australia. |
MSc | 2018 |
Coastal and Marine Engineering and Management, Delft University of Technology (TU Delft), Netherlands |
BSc (Eng) | 2016 |
Harbour, Coastal and Offshore Engineering, Hohai University, China |
Dr. Yongjing Mao (preferred name: Mao) is an Associate Lecturer at the Water Research Laboratory within the School of Civil and Environmental Engineering. Mao holds a Bachelor's and Master's degree in Coastal Engineering and a PhD in Remote Sensing. Prior to his position at UNSW, he worked as a Postdoctoral Research Fellow at the University of Queensland.
Mao is an expert in integrating coastal morphology knowledge with remote sensing and machine learning methods to understand shoreline changes and predict future states at regional, continental, and global scales. His research interests include the application of remote sensing, deep learning, and data assimilation to shoreline monitoring and modelling, with a focus on improving satellite-derived shoreline mapping through SAR-Optical fusion, employing data assimilation for shoreline modelling, and using innovative deep learning spatio-temporal models to predict future shoreline changes.
- Publications
- Media
- Grants
- Awards
- Research Activities
- Engagement
- Teaching and Supervision
2022. UQ Dean's Award for Outstanding HDR Theses
2016. Erasmus Mundus Partner Country Scholarship (€49,000)
I apply spatio-temporal deep learning methods to model the shoreline change.
I extract shoreline from satellite images at regional, continental and global scales.
I study SAR-Optical satellite image fusion and explore its potential for shoreline monitoring.
I developed spatio-temporal deep learning models (e.g. ConvLSTM and PredRNN) for ground cover prediction in Great Barrier Reef Catchments.
I mapped coastal geomorphology on the global scale with the application of Google Earth Engine and machine learning.
My Teaching
CVEN9620 Rivers, Estuaries and Wetlands
ENGG2500 Fluid Mechanics for Engineers