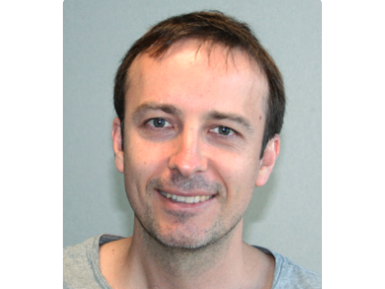
Associate Professor Pierre Lafaye de Micheaux
⇥ More details on my Personal Web Page
ABOUT ME
Biography
I have been an Associate Professor of Data Science and Statistics at the University of New South Wales since 2020. Previously, I was:
- Senior Lecturer of Statistics at the University of New South Wales (2017-2019);
- Professor of Statistics at ENSAI, the French National School of Statistics and Information Analysis (2015-2017);
- Associate Professor of Statistics at Université de Montréal, Department of Mathematics and Statistics (2011-2016);
- Assistant Professor of Statistics at Université de Montréal, Department of Mathematics and Statistics (2009-2011);
- Assistant Professor of Statistics at Grenoble Alps University, Laboratory Jean Kuntzmann (2003-present);
-
Affiliate Researcher at Grenoble Neuroscience Institute, in the Functional and Metabolic Neuroimaging team (2007-2010).
Education
- MSc in Cognitive Neuroscience, Grenoble Institute of Technology, France, 2007.
- PhD in Statistics,Université de Montréal and Université de Montpellier, Canada and France, 2003.
- MSc in Biostatistics, Université de Montpellier, France, 1998.
- BSc in Pure Mathematics and Physics, Université de Montpellier, France, 1996.
Training
- Postdoctoral Fellow, Brain Imaging Center, McGill University, Canada, 2003.
- Postdoctoral Fellow, Centre de Recherches Mathématiques (CRM), Canada, 2003.
RESEARCH
Research Goals
- Develop statistical tools that are used inside and outside our community;
- Use tools of Pure Mathematics to propose or improve new statistical methods;
- Build computer packages that permit the widespread of these new methods;
- Collaborate with researchers in Australia and Overseas.
Research in Detail
My main current research interests are in the four following inter-related topics.
- Dependence Measures: We plan to devise novel and easy to use statistical methods for better analyses of dependencies between random elements of various types, with emphasis on big data. Challenges arising in the big 3V's-environment (Volume, Variety, Velocity) will be mainly addressed by taking advantage of results from complex analysis. We plan to develop a unifying methodology that relies on the complex-valued characteristic function, which will allow dependence between any number of random elements, even big, to be apprehended.
- NeuroImaging Genetics: This new field of scientific research refers to the use of anatomical or physiological brain imaging technologies (fMRI, EEG, DTI) as phenotypic assays to evaluate genetic variation. I plan to develop new statistical methods, in close connection with neuroscientists (CHeBA, INSERM), to help to understand these very interesting problems.
- Big Data and Internet of Things: We are now overwhelmed with data. Moreover, big data sets typically involve mathematical objects much richer than simple random variables, hence showing relationships of much more intricate nature. These data can come in multiple forms, or as streams in real-time. The latter is particularly true for data recorded using sensors attached to physical devices. When all these objects are connected together through the Internet, they form an Internet of Things (IoT). Recently, a tiny and affordable computer that can be equipped with many cheap and various sensors, the Raspberry Pi, has gained popularity. I plan to develop new computing tools to control the Pi and propose new statistical tools to analyse data acquired using this credit-card size computer.
- Statistical Inference for Complex Random Vectors: Almost all current statistical modelling techniques are designed for real-valued data. Though, complex-valued random vectors are common in some important applications of big data. For instance, in functional magnetic resonance imaging (fMRI), the raw data returned by the scanner are complex numbers. For steam generator tubes in nuclear power plants, defects are periodically checked by means of complex Eddy-current probes measurements. It is thus important to build a complete theory of statistical inference for complex-valued random vectors.
Research Grants
- UNSW Research Infrastructure Scheme (2020-2021);
- NSERC individual competition discovery grant program (2014-2019);
- Mitacs Accelerate Research Internships Program (2013);
- NSERC Research Tools and Instruments Grants Program (principal investigator) (2013).
Current Student Projects (PostDoc, PhD and Honours)
- Rianti Siswi Utami, Ph.D. student (2020-), joint supervision with Prof. J. Olivier.
- Guillaume Boglioni-Beaulieu, Ph.D. student (2017-), joint supervision with Prof B. Avanzi and Prof B. Wong (from UNSW Business School).
Supervision Opportunities/Areas
I will be happy to supervise you in any specific areas in which you have an interest: both in theoretical/applied statistics, with any application you may have in mind (such as but not limited to: Big data, Neuroscience, Medicine, Internet of Things, etc.), and to develop computer software packages to promote your research.
Advice for Prospective Students
I am looking to work with enjoyable, hard-working, passionate, and autonomous students. Have a look at my Expertise, my Publication records, and my Personal web page to see the kind of things I have been interested in. Feel free to come and discuss any research related matter!
TEACHING & OUTREACH
Courses I Teach
Current
- ZZSC5905: Statistical Inference for Data
Recent years
- DATA3001: Data Sciences & Decisions in Practice, at UNSW Sydney
- MATH3821: Statistical Modelling and Computing, at UNSW Sydney
- MATH1041: Statistics for Life and Social Science, at UNSW Sydney
- MATH5806: Applied Regression Analysis, at UNSW Sydney
- MBDSTA02: Statistical Inference and Hypothesis testing, at ENSAI
- R/Shiny: Interactive Web Apps, at ENSAI
- MBDINF14: Programming with Big Data in R using Distributed Memory, at ENSAI
- STT1700: Introductory Statistics, at Université de Montréal
- STT2400: Linear regression, at Université de Montréal
- STT6415: Regression Analysis, at Université de Montréal
- STT2700: Mathematical Statistics and Data Analysis, at Université de Montréal
- STT6300: Large Sample Techniques, at Université de Montréal
Professional affiliations and service positions
- Editorial board, PratiqueR collection (French), Edition Diffusion Presse Sciences. 2013 - present.
- Editorial Board, Journal of Statistical Software. 2017 - present
Consulting to Industry, Commerce, and Government
I have provided consulting and contract research activities for BNP Paribas (Deep Learning techniques to predict credit default); Olea Medical (prediction of recanalization after thrombolysis for patients with acute stroke); Danone Research (study of benefits of probiotic fermented milk); Minvasys (sample size computation for a clinical study on drug eluting stents); etc.
AWARDS & ACHIEVEMENTS
- Université de Montréal Faculty of Advanced Studies Provost Honor List (2003).
My Expertise
Asymptotics, Big Data, Biostatistics, Bootstrap, Complex random fields, Data Science, Developing R packages, Hypothesis testing theory, Independent Component Analysis, Linear regression methods, Model selection, Multiple testing, Multivariate statistics, Neuroscience, Reproducible research, Sample size determination, Stochastic processes, Teaching, Time series analysis.
- Publications
- Media
- Grants
- Awards
- Research Activities
- Engagement
- Teaching and Supervision