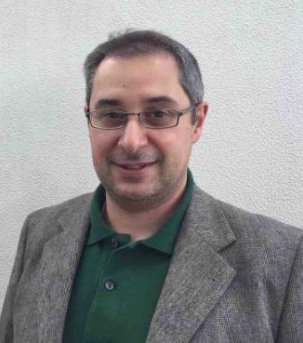
Dr Lorenzo Vigentini
Research Interests
My background is in Psychology, Learning and teaching in the Higher Education sector and have a lot of experience in IT/e-learning. My expertise is into learning processes at the crossing between cognitive psychology, differential psychology, education and human-computer interaction. My main interest is about technology, its use, its evolution, its interaction with learning and the interface between human and machines (also physical using computer vision and brain activity monitoring).
In the educational context this means a keen interest for the student experience considering specifically how learning technology and by technological innovation can support teaching excellence, augment Quality Assurance processes and aid Quality Enhancement.
However, to study the impact of technological innovation, I am a strong supporter of data-driven approaches to understand patterns and relations. In recent years this has been termed Learning Analytics or Educational Data Mining. Such an approach is essential to inform Institutional Research and evidence-driven practice providing a stronger perspective than traditional educational discourse. I am interested in MOOCs development and evaluation and the use of new 'smart' technologies as tools to support and enhance teaching and learning.
In the past few years I led the development of the Learning Analytics and Educational Data Science research group at UNSW.
I am verse with both quantitative and qualitative methods and I am always keen to learn about new methodologies from interdisciplinary cross-insemination.
Educational data mining is one of such examples I have been working with to explore emergent patterns in students' types from cognitive/learning styles, academic performance and interaction with learning technology. Sentiment analysis is another application of student generated data which the Universities gather but do not use much.
I am also interested in the cognitive, emotional and social determinants of performance under undue stress (e.g. students’ first year undergrad experience, work environment in highly competitive or critical situations, decision making processes or dysfunctional team work).
One more area attracting my interest is how individual differences shape team interaction and drive entrepreneurial behaviour and whicj sychometric markers are most important in determining entrepreneurial success.
Recent Publications
Vigentini, L., Swibel, B. & Hasler, G. (in publication). A comparison of LA dashboards in Schools and Higher Education: a focus on goal setting and support processes. In Ifenthaler, Muhittin (Eds.) Visualisations and dashboards for learning analytics.
Arthars, N., Dollinger, M., Vigentini, L., Liu, D., Kondo, E., & King, D. (2019). Empowering teachers to personalize learning support. In Ifenthaler, Mah, Yau (Eds.) Utilizing Learning Analytics to Support Study Success. https://www.springer.com/gp/book/9783319647913
Swibel, B., Hasler, G. & Vigentini, L. (under review). Developing a growth learning data mindset: a secondary school approach to creating a culture of data driven improvement. Journal of Learning Analytics, Special issue ‘Learning analytics in schools’
Vigentini, L., Liu, D. Y. T., Arthars, N., & Dollinger, M. (2020). Evaluating the scaling of a LA tool through the lens of the SHEILA framework: A comparison of two cases from tinkerers to institutional adoption. The Internet and Higher Education, 45, 100728. https://doi.org/10/ggm39d
Ford, R., Vigentini, L., Vulic, J., Chitsaz, M., Prusty, B.G., (2019) A Massive Open Online Course (MOOC) on Engineering Mechanics: Data Analytics Informing Learning Design and Improvement. Australian Journal of Mechanical Engineering https://doi.org/10.1080/14484846.2019.1596049 ,
Pardo, A., Bartimote, K., Shum, S. B., Dawson, S., Gao, J., Gašević, D., … Vigentini, L. (2018). OnTask: Delivering Data-Informed, Personalized Learning Support Actions. Journal of Learning Analytics, 5(3), 235–249–235–249. https://doi.org/10.18608/jla.2018.53.15
Vigentini L; Mirriahi N; Kligyte G, 2016, 'From reflective practitioner to active researcher: Towards a role for learning analytics in higher education scholarship', in Spector M; Lockee BB; Childress MD (ed.), Learning, Design, and Technology. An International Compendium of Theory, Research, Practice, and Policy, Springer International Publishing, pp. 1 - 29, http://dx.doi.org/10.1007/978-3-319-17727-4_6-1, ROS ID: 810326
Vigentini L; McIntyre S; Mirriahi N; Alonzo D, 2016, 'Exploring the real flexibility of learning sequences: Does course design constrain students behaviours or do students shape their own learning?', in ElAtia S; Zaïane O; Ipperciel D (ed.), Data Mining and Learning Analytics in Educational Research, Wiley and Blackwell, ROS ID: 511538
Chitsaz, M., Vigentini, L., & Clayphan, A. (2016). Toward the development of a dynamic dashboard for FutureLearn MOOCs: insights and directions. In 33rd International Conference of Innovation, Practice and Research in the Use of Educational Technologies in Tertiary Education (p. 116).
- Publications
- Grants
- Awards
- Research Activities
- Engagement
- Teaching and Supervision
- Media
- 2019 – UNSW DVCA: Biometrics in Learning & Teaching and VR – AUD 85,000
- 2019 – UNSW Faculty Seed Grant: The Experience of Information systems/Computing Science students. Co-led with Lesley Land (School of Information Systems) – AUD 10,000
- 2018 – UNSW ‘Students as Partners’; Program co-lead in the PVCE – AUD 1.2M
- 2016 – Office of Learning & Teaching SP16-5264 (Strategic Priority Projects) 'Scaling the provision of personalised learning support actions for large student cohorts', co-investigator - AUD 350,000
- 2016 – UNSW PVC Education ‘Heroes Program’ fund (project designed to extend the students as partners’ program) – AUD 190,000
- 2015 – UNSW Learning and Teaching Unit ‘Skunkworks’ fund (project designed to develop sprints of development in Learning analytics and Educational Data Science in partnership with students) – AUD 120,000
- 2013 – The Division of the DVC Academic, Student Voice Project (An evaluation and redesign of the Course And Teaching Evaluation Instrument), Principal Investigator - AUD 36,000
- 2012 - Higher Education Academy, An Evaluation of the PRES (Postgraduate Research Experience Survey), Principal Investigator – GBP 11,500
- 2007-08 – College of Humanities and Social Sciences - Conference Travel Grant – GBP 1,000
- 2004-06 - Co-researcher for Psychology in the STEER Project - The University of Edinburgh Principal E-learning Fund – GBP 69,000