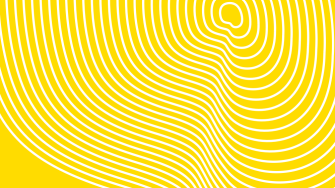
Objectives & Deliverables:
- Collection of industrial data to train a NN for making future demand predictions and increasing its accuracy by fine-tuning its hyper-parameters. This involves collecting suitable time series data and developing a suitable machine learning model to make future predictions. The model can be a combination of different algorithms and fine-tuned and adjusted accordingly so that it is capable of better predictions than some models in current literature.
- Implementation of the forecasting model on some suitable sectors of the supply chain (mentioned previously) to minimise supply chain cost. Current supply chains are very complex and intricately interconnected. A neural network can help understand the interrelationship between various sectors and processes in the chain and can help in reducing the overall cost of the chain. By predicting the future demand, the delivery performance, customer satisfaction, and inventory management can be efficiently performed, and the overall cost KPI (Key Performance Indicators) can be improved.
- Integration of TL algorithm with the existing NN model in order to evaluate how knowledge gained from one sector of the supply chain can be applied to make future predictions on other similar sectors. Some critical agents in a supply chain network are retailers, warehouses, distributors, and manufacturers. Identifying similar tasks and the level of similarity among them can be used to analyses the significance of TL on the whole chain. Using TL, whether agents have the same network structure or not, while sharing the learned network among them can also be an interesting case to study.
Project start date: 19 October, 2019.
Expected completion date: 13 March, 2023.
Achievements:
Ahmed, S., Chakrabortty, R. K., Essam, D.L., Ding, W. (2021) “Poly-linear Regression with Augmented Long Short Term Memory Neural Network: Predicting Time Series Data”, Information Sciences, (IF: 6.795, Q1). (Submitted, under review)
Ahmed, S., Chakrabortty, R. K., Essam, D.L., Ding, W. (2022) “A Switching Based Forecasting Approach (SBFA) for Forecasting Sales Data in Supply Chains”, Neurocomputing, (IF: 5.719, Q1). (Submitted, under review)
Student: Supriyo Shafkat Ahmed
Supervisors: Daryl L Essam and Ripon K Chakrabortty

Key contact
Dr Ripon K. Chakrabortty, opens in a new window
M: +61 414 388 209
E: r.chakrabortty@unsw.edu.au