Artificial Intelligence for Decision Making
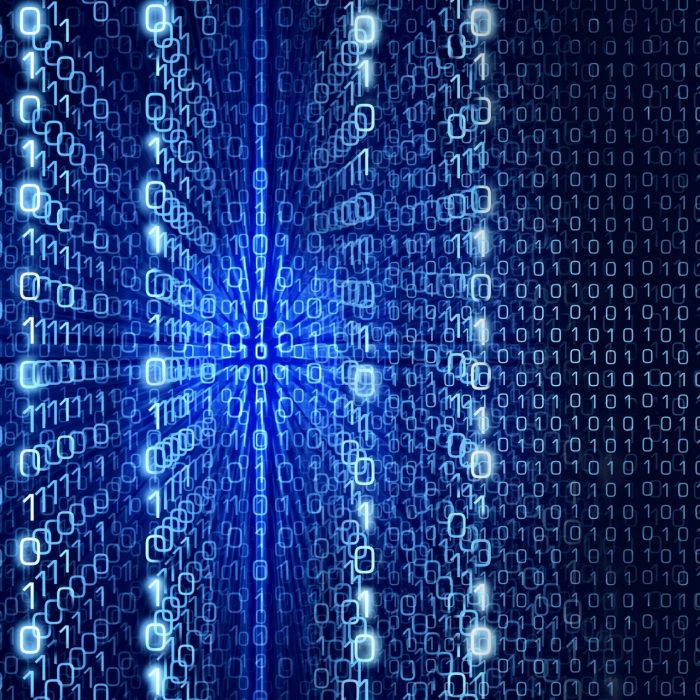
Data-driven AI in decision making
Notably, advancements in computing power and the requisite for real-time prediction and decision-making by analysing large-scale data have caused AI proliferation in the last few decades, which are very few in the supply chain and project management domains. Integrated project planning and scheduling is a popular study area that has offered a blueprint for project success based on on-time completion. However, in real-world production, the project environment changes constantly due to external and internal factors like machine breakdowns, sudden material shortages, and so on, causing process pauses. As a result of these disruptions, the best process plan and timetable may become less efficient or even impossible to implement. In reality, each project is vulnerable to a wide range of hazards that might stymie development or result in significant quantitative or qualitative harm. As a result, project risk management has evolved into an important aspect of project management that entails predicting, analysing, and controlling project risks while planning and controlling risk to reduce the negative impact of any risk on project results. In terms of project risk management entails the analysis of large amounts of project data and the decision-making and planning processes that result in additional time and money. Project risk management systems may significantly reduce risk management expenses while also acting as a decision support system. Artificial and Data Intelligence Technology will have a growing impact on projects, and in particular, project risk management. While no one knows exactly how Artificial Intelligence (AI) will affect project risk management, almost everyone agrees that it will. According to statistics, AI will most certainly automate many administrative project management duties. "AI will construct first draught programme schedules and risk registers using hard facts," for example. Furthermore, whereas conventional project management centred on activities such as scheduling and tactical planning, AI will likely automate much of that work, changing the Project Manager's primary attention to figuring out how to use this technology to provide greater value to consumers. However, most AI-based risk management systems are confined to a few risk categories, limited data features, and small datasets, making them unworkable for real-world project settings. Therefore, this research group has been creating different AI-based risk management systems for complex projects.
Similarly, today's supply chain businesses aren't operating as normal. As technologies like digital twins, machine learning (ML), and the internet of things (IoT) mature and spread, businesses worldwide will be able to achieve things they never could before. These advanced technologies can help firms establish an intelligent supply chain that forecasts and monitors the impact of practically every action they make, allowing them to balance three critical goals (i.e., relevance, resilience and responsibility) that supply chain professionals are expected to achieve on a continuous and simultaneous basis. Our research group has demonstrated that applications of AI in supply chain resilience (SCR) can contribute to accurate results in different aspects, including, but not limited to, ripple effect modelling, identifying and predicting risks and uncertainties, and data-driven planning of the system. However, industry stakeholders are still largely uninformed of AI's potential, particularly in terms of risk and uncertainty management, since the use of these techniques for SCR management is still in its infancy. Hence, we, as a group, have been proposing different advanced and hybrid AI-based approaches, which are being applied to supply chain, project management, feature extractions, and other predictive analytic techniques.
Explainable AI in decision making
Although AI models’ numerous qualities typically help them achieve higher performance, their complexity often makes their basic working principle difficult to comprehend. This lack of readability and clarity inevitably raises questions about their reliability and impartiality. Regardless
of their accuracy, this effectively questions the classifiers’ reasoning and supports the mistrust. The bulk of existing state-of-the-art AI models has comparable flaws, prompting the development of eXplainable AI (XAI), aiming to create approaches that can improve the interpretability and explainability of AI models. The primary purpose of XAI is to create a simple representation of any AI model that can help humans comprehend the model’s conclusion in relation to any given
input using logical or conceptual reasoning. This is sometimes referred to as a model’s interpretability. Because the AI models’ predictions and judgments may have a significant socioeconomic influence, subsequent regulations and concerns have made it critical to assure their legitimacy and rationality by giving easy-to-understand explanations to stakeholders. The development of acceptable explainable AI (XAI) approaches for risk management has become vital, given the bulk of AI techniques now employed in project risk management lack explainability. Despite recent advances in XAI methodologies, this discipline still relies significantly on domain expertise when it comes to communicating explanations to stakeholders. Some of the most extensively used AI models in project risk management have hardly been adopted to improve explainability. Developing appropriate XAI methodologies for real-world project scenarios may successfully give insights into the variables that influence project risks and make the project environment accessible to stakeholders, allowing them to plan preventive maintenance and budget accordingly. As a result, we are working to close the research gap in developing acceptable XAI approaches for intelligent risk management systems for both supply chain and project management problems.
Impact
Our research is aimed at delivering:
- Advanced and hybrid AI-based approaches for predictive analytics
- Tools to proactively assess a supply chain network’s ability to mitigate the impact of future disruptions
- An efficient marriage of machine intelligence and human quotients to design reliable and integrated decision support tool
- Augmented AI tools by hybridising advanced single- and multi-objective optimisation techniques.
Competitive advantage
Our research group bring together experts with remarkable track records in Cross-disciplinary research areas (such as supply chain management, network science, evolutionary algorithms, artificial intelligence business modelling, and simulation & modelling). We have long-standing experience in working with different project scheduling problems and then, consecutively, solving them by different advanced evolutionary algorithms. We can help organisations to better understand their capacity system and how it may lead to enhanced outputs and results, starting with the assumption that the focus should be on achieving high performance. Our emphasis on collaborating with organisations and doing applied research helps practitioners get the information and skills they need to put our research results into practice. The research team has extensive experience designing and implementing funded research from the ARC, industry partners, and other sources. We ensure that for any potential project design, the composition of the research team and the budget required for that project will reflect a concerted effort to calibrate a project that accounts for common project risks. In addition, we endeavour to communicate our findings to the public using local and national online news and communication platforms such as LinkedIn and other social media. Through UNSW, this research group has access to the supercomputer at National Computational Infrastructure (NCI), which is used to develop and test the developed algorithms. We have the necessary office space, access to information and library resources necessary to carry out any possible research matters.

Key contact
Dr Ripon K. Chakrabortty, opens in a new window
M: +61 414 388 209
E: r.chakrabortty@unsw.edu.au